Making the Most of Small Data in Scientific R&D
For many traditional innovation-driven organizations, scientific data is generated to answer specific immediate research questions and then archived to protect IP, with little attention paid to the future value of reusing the data to answer other similar or tangential questions. Data is essentially a side product of R&D and not viewed as a primary output. As a result, important experimental process details and implied contextual information are often not recorded.
Data that is collected is often not formatted in a consistent and well-structured manner, making it difficult and expensive to parse large volumes of historical data files that may be archived in a network drive or data lake. And the experimental workflows that produce this data are typically manual and require coordination between multiple teams—manual sample preparation and handoff between labs, manual data transfer between computers, manual raw data analysis on instrument computers. All these challenges make new data generation very slow and expensive.
The result is that many R&D labs have surprisingly small datasets that are actually clean enough and complete enough to serve a higher purpose, like as training data for a machine learning model.
Faced with their “small data” situation, researchers and managers often feel that they may not yet benefit from pursuing data-driven approaches to new product development. They are not sure what can be done given the current state of their data or how to efficiently gather more data to alleviate the issue. Even in organizations that have pushed forward a high-level vision with one-size-fits-all data platforms, new data science and engineering teams struggle to generate value due to the unique challenges inherent to scientific small data problems.
At Enthought, we have tackled many small data challenges in science-driven product development and have employed multiple strategies for getting the most value out of our customers’ small data to meet their strategic innovation goals. While there is no universal solution because each R&D organization has unique data and workflows, we help make the most of what they have and set a course towards continuous improvement. Teams can actually get started with little to no data and leverage existing domain knowledge to get further with less data through well-crafted experimental designs, feature engineering, informed model constraints and priors, and improved data quality. We also assess existing data generation workflows and prioritize workflow improvements that will accelerate new data generation and improve data quality using software tools to streamline data labeling tasks and to automate or assist users with raw data analysis.
Do you have a small data challenge in your lab?
Don’t let that stop you from getting started with data-driven methods. In fact, it is in the organizations where small data is the norm where data-driven modeling and prediction can provide the most value and accelerate discovery and innovation.
Contact us today to discuss your team's small data challenges.
Enthought at AIChE 2023
Attending the American Institute of Chemical Engineers (AIChE) Spring Meeting in Houston? Come join Enthought’s Material Informatics expert Dr. Michael Heiber for an in-depth discussion about making the most of small data in materials science and chemistry research on Wednesday, March 15, 2023.
About the Author
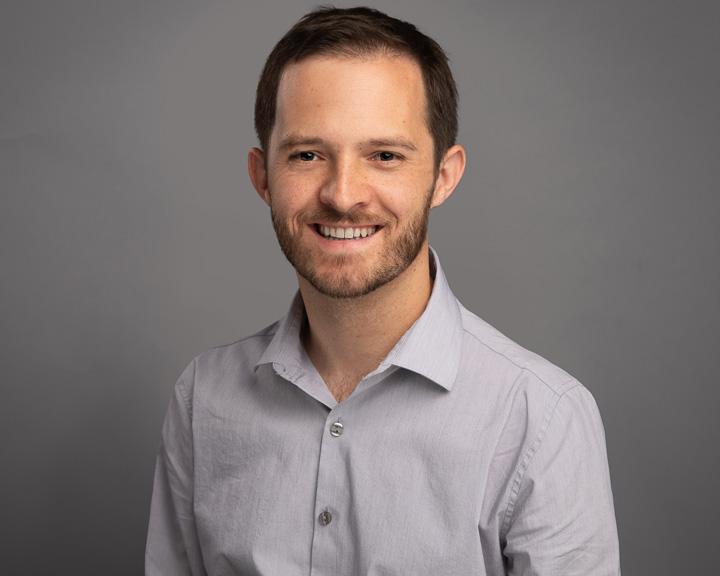
Michael Heiber holds a Ph.D. in polymer science from The University of Akron and a B.S. in materials science and engineering from the University of Illinois at Urbana-Champaign with expertise in polymers for optoelectronic applications. At Enthought, he leads the Materials Informatics Team helping clients leverage machine learning and AI to make better, faster R&D decisions.
Prior to joining Enthought, he worked as a postdoctoral researcher at several institutions, where he worked to digitally transform organic electronic materials and device development using physics-based simulations, automated experimental measurements, and automated data analysis tools. At Enthought, Michael has utilized these diverse experiences in the Materials Science Solutions Group to help accelerate and transform industrial materials R&D with several key clients. He now oversees the Materials Informatics Team and Materials Informatics Acceleration Program at Enthought.
Related Content
Digital Transformation vs. Digital Enhancement: A Starting Decision Framework for Technology Initiatives in R&D
Leveraging advanced technology like generative AI through digital transformation (not digital enhancement) is how to get the biggest returns in scientific R&D.
Digital Transformation in Practice
There is much more to digital transformation than technology, and a holistic strategy is crucial for the journey.
Leveraging AI for More Efficient Research in BioPharma
In the rapidly-evolving landscape of drug discovery and development, traditional approaches to R&D in biopharma are no longer sufficient. Artificial intelligence (AI) continues to be a...
Utilizing LLMs Today in Industrial Materials and Chemical R&D
Leveraging large language models (LLMs) in materials science and chemical R&D isn't just a speculative venture for some AI future. There are two primary use...
Top 10 AI Concepts Every Scientific R&D Leader Should Know
R&D leaders and scientists need a working understanding of key AI concepts so they can more effectively develop future-forward data strategies and lead the charge...
Why A Data Fabric is Essential for Modern R&D
Scattered and siloed data is one of the top challenges slowing down scientific discovery and innovation today. What every R&D organization needs is a data...
Top 5 Takeaways from the American Chemical Society (ACS) 2023 Fall Meeting: R&D Data, Generative AI and More
By Mike Heiber, Ph.D., Materials Informatics Manager Enthought, Materials Science Solutions The American Chemical Society (ACS) is a premier scientific organization with members all over…
Real Scientists Make Their Own Tools
There’s a long history of scientists who built new tools to enable their discoveries. Tycho Brahe built a quadrant that allowed him to observe the…
How IT Contributes to Successful Science
With the increasing importance of AI and machine learning in science and engineering, it is critical that the leadership of R&D and IT groups at...
From Data to Discovery: Exploring the Potential of Generative Models in Materials Informatics Solutions
Generative models can be used in many more areas than just language generation, with one particularly promising area: molecule generation for chemical product development.