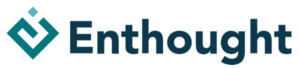
Deep Learning for Scientists & Engineers
Gain a practical introduction to deep learning using Keras and TensorFlow with focus on the fundamental model architecture: the neural network.
Course Overview
Deep Learning for Scientists and Engineers provides students with a practical introduction to deep learning using Keras and TensorFlow.
This course focuses on the fundamental model architecture used in deep learning: the neural Network.
We will begin by building a solid foundation of the basics of deep learning and will gradually progress into more advanced topics like model training and Evaluation.
While the course emphasizes a practical approach to deep learning, there are times where just enough theory is covered to understand the why behind certain modeling procedures.
Prerequisite
This course requires basic proficiency with Python and the scientific Python stack. Some practical experience with Jupyter Notebooks, NumPy (ndarrays), Pandas (DataFrames), and scientific visualization in Python using Matplotlib are essential to working with the code and concepts presented in this course.
If you have taken Enthought’s Python Foundations for Scientists and Engineers, you have the requisite background knowledge for this course. While not a strict requirement, it is strongly recommended to have taken Enthought’s Machine Learning for Scientists and Engineers (or have a working knowledge of basic machine learning principles) prior to taking this course.
Lectures
Intro to Deep Learning & Neural Networks – Artificial Intelligence (AI), Machine Learning (ML)
Keras & Tensorflow – Neural Network Architecture (Layers & Activation Functions)
Building Neural Networks – Sequential Model, Compiling & Fitting Models
Tuning Neural Networks – Data Partitioning, Model Learning History
Saving & Loading Models – Model Checkpointing, Transfer Learning
Training Neural Networks I – Automatic Differentiation
Training Neural Networks II – Custom Training Loops
Custom Reporting – Keras Callbacks
Evaluating Neural Networks I – Evaluation Metrics, Diagnostics & Remedies
Evaluating Neural Networks II – Model Appraisal
Packages
tensorflow
Download the syllabus for this course here.
Updated December 2023