Data-Centric Deep Learning
The dominant paradigm for utilizing AI is model-centric—you fix the data you're working with and you tweak your model to get a good fit. There has been a recent shift towards data-centric AI—focus on data quality once you have a reasonable model. We recently applied this approach for an AI-driven microscope we're developing, and improved model accuracy and eliminated a lot of model code. That was very satisfying!
The data-centric approach aligns very well with how we approach scientific data in general. Scientists use instrumentation, measurement, and analysis to understand how materials properties affect performance—those properties are features. When your measurements and analysis are inconsistent, your features aren't going to be that useful for machine learning or anything else. (We built the microscope to address this problem, which sped up data collection and reduced human error.) Whether it's AI or laboratory transformation, you should ask yourself, "What is the quality of my data and how do I go about improving it?"
Sustainability and Decarbonization
These are not new topics, but they really came to life in 2022. We are seeing large sustainability and decarbonization investments being made at multiple levels across the materials, semiconductor, and energy industries. Materials and Energy companies are elevating the performance targets of their energy materials and technologies, and developing new products like catalysts for carbon utilization. Development of battery materials is particularly intense. We understand why; Bloomberg says to expect 15x market growth by 20303. At SEMI SMC this year we learned about multiple climate and sustainability pledges being made by industry leaders (Intel, Micron, others). Related, SEMI recently announced the Semiconductor Climate Consortium4. (We're happy to see multiple Enthought clients among the founding members!) In Japan, home to many of our clients, METI has established the GX League5 and is calling on all companies to consider how to spur economic growth through green transformation.
Traditional, slower discovery methods won’t be adequate to solve these big challenges as fast as we need. Digital transformation is going to be an enabler, if not a necessity, of innovation in this space. As a team of data-centric scientists and engineers, we find this all very motivating. Many people who go into science do so because we want to make a lasting impact on the world. At Enthought we harness that drive in order to help our industrial clients transform how they do science. When that local impact aligns with a trend towards the greater good, it reminds us of why we love what we do!

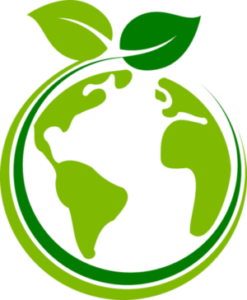
Contributors:
- Chris Farrow, Ph.D., Vice President, Materials Science Solutions | Chris currently leads Enthought’s Materials Science Solutions Group, where he oversees Digital Transformation solutions for Specialty Chemicals and Semiconductor industries. He holds a Ph.D. in physics from Michigan State University and degrees in physics and mathematics from the University of Nebraska.
- Tim Diller, Ph.D., Director, Digital Transformation Services | Tim loves working at the intersection of technology, commerce, and human organization. He holds three degrees in mechanical engineering, including a Ph.D. and B.S. from The University of Texas at Austin and an M.S. from M.I.T.
- Behzad Eftekhari, Ph.D., Consulting Manager | Behzad has a passion for applied, physics-based solutions. He holds a Ph.D. in petroleum engineering from the University of Texas at Austin, and a BSc in mechanical engineering from the Sharif University of Technology in Iran.
References
Related Content
Digital Transformation vs. Digital Enhancement: A Starting Decision Framework for Technology Initiatives in R&D
Leveraging advanced technology like generative AI through digital transformation (not digital enhancement) is how to get the biggest returns in scientific R&D.
Digital Transformation in Practice
There is much more to digital transformation than technology, and a holistic strategy is crucial for the journey.
Leveraging AI for More Efficient Research in BioPharma
In the rapidly-evolving landscape of drug discovery and development, traditional approaches to R&D in biopharma are no longer sufficient. Artificial intelligence (AI) continues to be a...
Utilizing LLMs Today in Industrial Materials and Chemical R&D
Leveraging large language models (LLMs) in materials science and chemical R&D isn't just a speculative venture for some AI future. There are two primary use...
Top 10 AI Concepts Every Scientific R&D Leader Should Know
R&D leaders and scientists need a working understanding of key AI concepts so they can more effectively develop future-forward data strategies and lead the charge...
Why A Data Fabric is Essential for Modern R&D
Scattered and siloed data is one of the top challenges slowing down scientific discovery and innovation today. What every R&D organization needs is a data...
Top 5 Takeaways from the American Chemical Society (ACS) 2023 Fall Meeting: R&D Data, Generative AI and More
By Mike Heiber, Ph.D., Materials Informatics Manager Enthought, Materials Science Solutions The American Chemical Society (ACS) is a premier scientific organization with members all over…
Real Scientists Make Their Own Tools
There’s a long history of scientists who built new tools to enable their discoveries. Tycho Brahe built a quadrant that allowed him to observe the…
How IT Contributes to Successful Science
With the increasing importance of AI and machine learning in science and engineering, it is critical that the leadership of R&D and IT groups at...
From Data to Discovery: Exploring the Potential of Generative Models in Materials Informatics Solutions
Generative models can be used in many more areas than just language generation, with one particularly promising area: molecule generation for chemical product development.