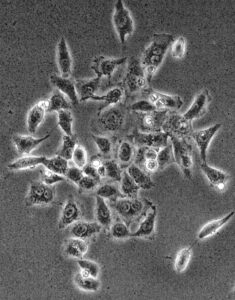
HEK 293 cells grown in tissue culture medium.
Mammalian cell culture is a fundamental tool for many discoveries, innovations, and products in the life sciences. Currently, cells are the smallest unit of sustainable life outside the body, thereby providing an essential platform for testing hypotheses and mimicking biological processes. The applications of cell culture, while not limitless, are plentiful.
Every cell type, downstream application, and use case is somewhat unique. With each new application, a laboratory team must learn, sometimes laboriously, the characteristics of the cell type and redefine optimal growth conditions or purchase the rights of a proprietary method, if it exists. The protein-producing machinery of some cell types can be harnessed to generate large quantities of therapeutic proteins. The complex, diseased state of primary tumor cells might be used to evaluate the response to drug compounds and perhaps even find a unique biomarker “signature” to explain an overly positive or negative result. Some immortalized cells are stored for later use in a quality control assay. Other cell types may be used to quantify the genomic, transcriptomic, and proteomic in response to external stimuli.
Traditional Workflows Limit the Rate of Discovery
During cell culture analysis, each time a visual inspection is performed under the microscope, observations, and judgments are made about the cell and culture as a whole.
- Are there contaminants in the media?
- Are the cells stressed, perhaps as indicated by granules?
- What is the density of the cells in the culture dish (e.g. are they overcrowded)?
- What is the morphology of the cell, and is it consistent with expectations?
- Is it ready for the next step in the protocol?
Any of these visual observations can affect the cells and therefore the experiment. The uniqueness of each cell type and its associated experiment requires that the judgments be consistent and repeatable for optimal results.
While a myriad of hardware exists to automate the physical manipulation processes, there is still a heavy reliance on manual visual observations in the traditional lab. Each scientist requires extensive hands-on training from experienced senior scientists working with that particular cell in order to make correct judgements and decisions. This reliance on senior expertise and manual methods creates bottlenecks and limitations to discovery.
As we all know, time is market share in the highly competitive pharmaceutical industry, but most labs today still have a traditional, human-centric approach to research. For companies focused on one application, scientists work on a specific cell type and experiment day in and day out, developing expertise and intuition slowly over time. What about companies exploring multiple cell types, or R&D organizations looking to add a new capability? Senior scientists must first learn each new cell and process, then train their team members, creating additional strain on their own time while further compounding the slower pace of innovation.
Automated Decision-Making Streamlines Processes and Accelerate Analysis
With advanced technologies like artificial intelligence and machine learning, labs can now employ automated decision-making tools that can not only support cell culture analysis but streamline it as well. Instead of a largely manual, human-driven process, a purpose-built application allows the organization to evolve with their cells efficiently and effectively, and in a way that different methods can be easily applied across cell types.
In this modern environment, integrated AI and machine learning tools automate the lab and reduce the burden on researchers, material waste, and the subjectivity of the process. The process becomes much more scalable, exponentially extending the capabilities of the team.
Instead of a scientist learning and maintaining ten different cell types, each one of them being a little different from the last, that same scientist now has this machine learning tool acting as their eyes and ears. Instead of senior scientists spending weeks or even months training junior scientists on a specific cell type, trained models are available and usable by anyone in the laboratory, even the greenest scientists. And if an experienced scientist departs, taking years of knowledge and expertise with them, the established models ensure there is no impact on the quality and consistency of the established process. The same models can be used as a starting point for new cell types and studies.
How can AI automate your lab?
Cell culture has the potential to support innovation in countless ways, and the application of AI to cell culture analysis offers meaningful change to the scientists, the lab, and the company. Imagine the possibilities if you strategically leveraged AI and machine learning beyond cell culture analysis and throughout your lab and your team.
Enthought has extensive experience in optimizing R&D labs with tailored digital solutions for science-driven companies of all sizes and stages, from Fortune 500 to startups. Contact our team of experts today to see how we can address your challenges and advance your team’s work.
Related Content
Reshaping Materials R&D: Navigating Margin Pressure in the Specialty Chemicals Industry
The era of the AI Co-Scientist is here. How is your organization preparing?
The Emergence of the AI Co-Scientist
The era of the AI Co-Scientist is here. How is your organization preparing?
Understanding Surrogate Models in Scientific R&D
Surrogate models are reshaping R&D by making research faster, more cost-effective, and more sustainable.
R&D Innovation in 2025
As we step into 2025, R&D organizations are bracing for another year of rapid-pace, transformative shifts.
Revolutionizing Materials R&D with “AI Supermodels”
Learn how AI Supermodels are allowing for faster, more accurate predictions with far fewer data points.
What to Look for in a Technology Partner for R&D
In today’s competitive R&D landscape, selecting the right technology partner is one of the most critical decisions your organization can make.
Digital Transformation vs. Digital Enhancement: A Starting Decision Framework for Technology Initiatives in R&D
Leveraging advanced technology like generative AI through digital transformation (not digital enhancement) is how to get the biggest returns in scientific R&D.
Digital Transformation in Practice
There is much more to digital transformation than technology, and a holistic strategy is crucial for the journey.
Leveraging AI for More Efficient Research in BioPharma
In the rapidly-evolving landscape of drug discovery and development, traditional approaches to R&D in biopharma are no longer sufficient. Artificial intelligence (AI) continues to be a...
Utilizing LLMs Today in Industrial Materials and Chemical R&D
Leveraging large language models (LLMs) in materials science and chemical R&D isn't just a speculative venture for some AI future. There are two primary use...