There’s a long history of scientists who built new tools to enable their discoveries.
Tycho Brahe built a quadrant that allowed him to observe the path and distance of a comet as it crossed the solar system, helping to prove the heliocentric model of the way the stars and planets move. Galileo Galilei built his own telescope to study the night sky. Antoni van Leeuwenhoek built microscopes to study microbes. Marie Curie built ionization chambers to discover radioactivity. Rosalind Franklin built X-ray cameras to study DNA, viruses, and carbon. Nikola Tesla developed his eponymous coil to study X-rays, lighting, wireless power transmission, and phosphorescence.
For all of these scientists, their tools were not merely accessories to their work. The tools they built were the basis for discovery; they were central to science.
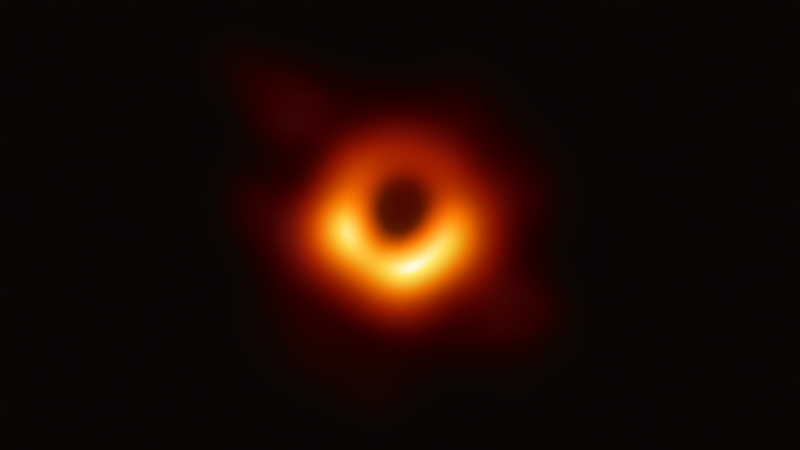
Event Horizon Telescope image of the M87 black hole.
Contemporary scientists still build physical tools, from tiny syringes to inject DNA into millimeter-long worms to gigantic participle accelerators. Still, they also require software and computational capabilities to enable discovery. Katie Bouman’s algorithm, and the contributions of other members of the Event Horizon Telescope Collaboration, which led to the first image of a black hole is a great example.
Yet, in the corporate world, we see that companies rely on machines built and software written by other companies. We’ve encountered scientists who use some metrics not because they’re good but because the software can compute them.
Many organizations attempt to solve this tool problem by assembling a team of software developers or data scientists to build the scientists’ ideas. In our experience, it does not work. The idea is “lost in translation” because developers lack the domain knowledge to understand the problem and build an appropriate solution. Or the idea is too fragile and dies because of the translation. The feedback loop is too slow in both cases to converge to a viable solution.
In the 21st century, enabling scientists to build their own tools often means enabling them to write their own software. If you’re a scientist, take control of your future and learn to program. If you’re the developer of a scientific application, always provide an “escape hatch” where scientists can access the raw data and make their own analyses. It’s impossible to anticipate everything they will need. A potent escape hatch is something like an embedded IPython prompt that gives people access to the raw data and objects without requiring an export. Data exports in standard formats are a bare minimum. Anything else will slow you down.
Don’t settle for the tools that you have. If you must, build the tools you need for your science.
Acquire the skills to build your own tools!
Machine learning algorithms share common scientific aspects with the process of building tools for discovery in scientific research. The open-source culture of machine learning practitioners is similarly collaborative to the scientific community, where scientists embrace peer review and collective learning.
Register for your seat in the upcoming course in Machine Learning, with Enthought Academy.
Author: Alexandre Chabot-Leclerc, Vice President, Digital Transformation Solutions, holds a Ph.D. in electrical engineering and a M.Sc. in acoustics engineering from the Technical University of Denmark and a B.Eng. in electrical engineering from the Université de Sherbrooke. He is passionate about transforming people and the work they do. He has taught the scientific Python stack and machine learning to hundreds of scientists, engineers, and analysts at the world’s largest corporations and national laboratories. After seven years in Denmark, Alexandre is totally sold on commuting by bicycle. If you have any free time you’d like to fill, ask him for a book, music, podcast, or restaurant recommendation.
Related Content
Leveraging AI for More Efficient Research in BioPharma
In the rapidly-evolving landscape of drug discovery and development, traditional approaches to R&D in biopharma are no longer sufficient. Artificial intelligence (AI) continues to be a...
Utilizing LLMs Today in Industrial Materials and Chemical R&D
Leveraging large language models (LLMs) in materials science and chemical R&D isn't just a speculative venture for some AI future. There are two primary use...
Top 10 AI Concepts Every Scientific R&D Leader Should Know
R&D leaders and scientists need a working understanding of key AI concepts so they can more effectively develop future-forward data strategies and lead the charge...
Why A Data Fabric is Essential for Modern R&D
Scattered and siloed data is one of the top challenges slowing down scientific discovery and innovation today. What every R&D organization needs is a data...
Jupyter AI Magics Are Not ✨Magic✨
It doesn’t take ✨magic✨ to integrate ChatGPT into your Jupyter workflow. Integrating ChatGPT into your Jupyter workflow doesn’t have to be magic. New tools are…
Top 5 Takeaways from the American Chemical Society (ACS) 2023 Fall Meeting: R&D Data, Generative AI and More
By Mike Heiber, Ph.D., Materials Informatics Manager Enthought, Materials Science Solutions The American Chemical Society (ACS) is a premier scientific organization with members all over…
Real Scientists Make Their Own Tools
There’s a long history of scientists who built new tools to enable their discoveries. Tycho Brahe built a quadrant that allowed him to observe the…
How IT Contributes to Successful Science
With the increasing importance of AI and machine learning in science and engineering, it is critical that the leadership of R&D and IT groups at...
From Data to Discovery: Exploring the Potential of Generative Models in Materials Informatics Solutions
Generative models can be used in many more areas than just language generation, with one particularly promising area: molecule generation for chemical product development.
7 Pro-Tips for Scientists: Using LLMs to Write Code
Scientists gain superpowers when they learn to program. Programming makes answering whole classes of questions easy and new classes of questions become possible to answer….