Public Courses in the Enthought Academy Scientific Python Curriculum
Announcing a New Partner for Public Courses
Enthought is dedicated to delivering digital transformation through integrated scientific solutions. With over two decades of experience, we've been leaders in scientific computing and digital transformations, driving organizational change across Life Sciences, Material Sciences, Semiconductor, and Energy domains.
To further support our mission of accelerating science in R&D organizations around the world, we have made the decision to focus our business on our solutions and products that combine application development, scientific workflow re-engineering, and training.
As such, as of December 2023, the public courses in our scientific Python curriculum, which you have come to know through Enthought Academy, will no longer be offered at Enthought. We are however delighted to announce our new licensed provider of the Enthought Academy scientific Python curriculum: Diller Digital, LLC.
Our world-class training in scientific Python has been delivered to over 10,000 scientists and engineers. We want to thank everyone who has joined us in the classroom over the years.
If you’re still interested in all things digital and data when it comes to scientific research, we invite you to subscribe to our Linkedin newsletter where Enthought will continue to share our insights with the community.
World-class Training in Scientific Python
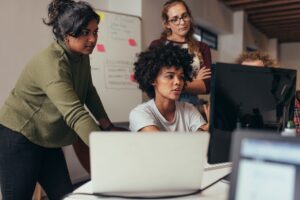
The Enthought catalog of world-class training programs is now available through our training partner:
Have a question for Enthought?
Send us a message by filling out our form and we will get back to you shortly.