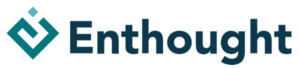
Machine Learning for Scientists & Engineers
Acquire skills in the basics of machine learning using problems drawn from science and engineering data sets. The course focuses primarily on supervised learning, regression, classification, and unsupervised machine learning strategies.
Course Overview
Machine Learning for Scientists and Engineers provides scientists and engineers with a practical introduction to classical machine learning using Scikit-learn.
The course focuses primarily on supervised learning, starting with regression and then moving into Classification.
Some unsupervised machine learning strategies will also be covered.
This course builds a solid foundation of the basics of machine learning, using problems drawn from science and engineering data sets.
Prerequisite
This course requires basic proficiency with Python and the scientific Python stack. Some practical experience with Jupyter Notebooks, NumPy (ndarrays), Pandas (DataFrames), and scientific visualization in Python using Matplotlib are essential to working with the code and concepts presented in this course.
If you have taken Enthought’s Python Foundations for Scientists and Engineers, you have the requisite background knowledge for this course.
Lectures
Introduction to AI/ML – Basic Terminology, Models
Introduction to scikit-learn – API, ML Workflow
Regression – Regression Models, Scoring
Feature Engineering I – Univariate & Bivariate Analysis
Feature Engineering II – Multivariate & Interaction Analysis
Feature Selection & Tuning – Regularization, Hyperparameters
Model Selection – GLM, SVM, Decision Trees, Ensemble
Classification I – Classification Models, Scoring
Classification II – Classification Workflow
Clustering – Algorithms
Packages
scikit-learn, seaborn
Download the syllabus for this course here.
Updated December 2023